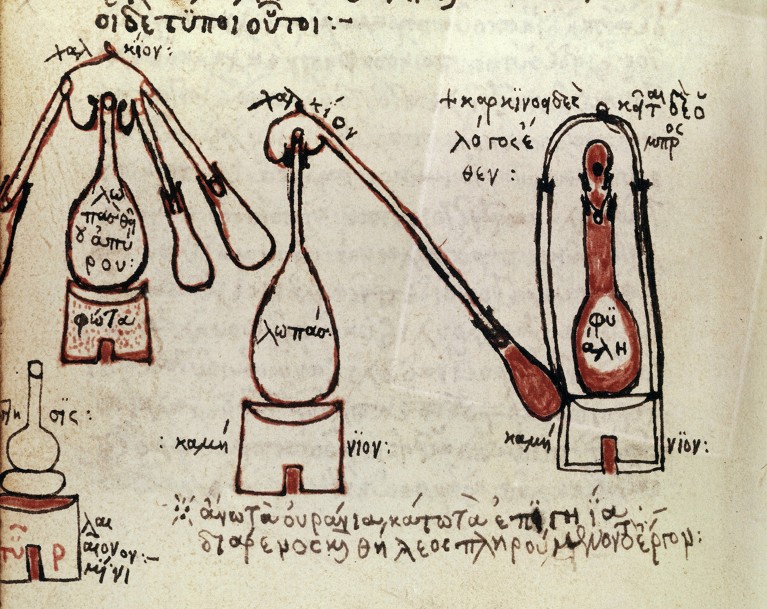
Jelle van der Hilst carefully plans her experiments on paper before heading to the bench, much like the alchemists who authored this 15th-century Greek manuscript.Credit: DeAgostini/Getty
When I decided to pursue a PhD five years ago, I was ready for the challenges of finding the right lab, securing funding, and designing the perfect project. I knew that analyzing and interpreting the results would produce difficult questions and sleepless nights, and I conscientiously reserved some mental capacity to worry about the eventual task of writing the thesis. Once I joined a laboratory in the Department of Bioengineering at the Massachusetts Institute of Technology in Cambridge, where I study the self-assembled proteins that form powerful lenses in the eyes of squid, I quickly realized that obtaining data would be much less difficult than interpreting it – or trusting it.
For example, I spent several weeks trying to measure the size of the protein particles I was working with to determine whether they self-assembled into larger aggregates. I applied the same technique to the same protein under the same conditions and obtained three very different results over three days, each supporting a different hypothesis. Data is data, I thought, until I had a pile of them and realized I had no idea if any of them were meaningful.
A perfectly executed experiment can produce completely inconclusive data, or you can get what looks like beautiful and exciting data from a botched experiment. How can you tell the difference? Below are some tips on how I learned to distinguish the two.
Balancing independence and mentorship
When I finally got my own project and bench as a PhD student, after years of hands-on lab work and closely supervised internships, I was desperate to do my own thing. I also thought that the doctoral students and postdoctoral researchers around me would be too busy with their own work to help me with mine, and that I had been hired to show that I could do independent work.
Collection: Scientific data
I started trying to put together protocols from various articles and fielding new lab equipment through trial and error. It took me five times longer to get data than if I had just had someone teach me the experiment in the beginning, and I never trusted the data – how could I to be sure I hadn’t missed a crucial step or a standard check, or that the protocols I had cobbled together were the most scientific approach? If I could go back in time, I would have a lab colleague teach me protein protocols the right way, from the beginning.
Be open about your results and feedback
I used to hide all my negative data and failed experiments. I wasn’t confident enough in my experiments to distinguish between the two, and I thought people would consider anything I produced that didn’t have a positive outcome a failure. Then I started casually mentioning the week’s frustration or bottleneck to anyone who would listen, and I always ended up getting some amazing advice, either for solving the problem or for knowing when to stop and move on. Regardless, it took me years to give up proteins that clearly didn’t work for their intended applications, even though everyone repeatedly told me to move on.
Postdocs and other more experienced researchers around you will have invaluable experience that could help you disentangle human error, background noise, and false positives or negatives from your actual experimental results. If nothing else, it might remind you that you’re trying to solve some really difficult problems.
Practice your protocols
No one excels at a new experience the first time. When I was first taught how to extract plasmid DNA from a bacteria, arguably one of the simplest protocols in my field, I think I missed every possible step – there are ten of them – at least once.
You won’t be able to trust your data until you trust your execution of the protocol, and it’s easy to make mistakes. Repeat the experiments on different days, with different reagents; don’t trust your first set of negative data; and don’t trust your first set of positive data either. When I obtained these widely divergent measurements of protein size, it was tempting to start with the first set of results, because they best matched my hypothesis. When I repeated the experiment with a more experienced hand, the results were disappointing – but I realized they were probably correct. Search is complicated and random errors can compound exponentially; Once you are confident that you have performed an experiment correctly, you can begin to trust what you see.
Separate planning from execution
Another factor in obtaining reproducible and reliable data is to ensure that your work is fully planned and verified before you begin. Yes, you can look up protocols on the fly or try to replace equipment if you realize a key machine is reserved a few minutes before you planned to use it, but even if you get data with these adaptations, a single mistake or shortcut can be fatal. uncertainty in the final results. I plan experiments from my desk, carefully adapt methods and print my own final protocols, then carry them out at my bench. Small improvisations can be impossible to remember and reproduce, and can lead to unpleasant surprises when you try to repeat the work later. Innocent shortcuts can also have unforeseen and unintended effects on science.
Learn to accept negative data
Finally, don’t let negative results keep you up at night: you can only control how you run the experiment, not what it tells you. Do your best, perform experiments with confidence, and share the results as honestly as possible. I was once told to imagine myself as an agnostic intermediary between science and my advisor; I can only report the findings that I observe, even if we would both prefer the data to be different.
Although in research it is crucial not to completely trust your data until it has been triple-proven and peer-reviewed, we must nevertheless gain some operational confidence in our methods and results. Otherwise, paralyzed by doubt, we would never bring any new research into the world.
This is an article from the Nature Careers Community, a place for Nature readers to share their professional experiences and advice. Guest posts are encouraged.
Competing interests
The author declares no competing interests.